All published articles of this journal are available on ScienceDirect.
A Novel Scale to Measure Nursing Students’ Fear of Artificial Intelligence: Development and Validation
Abstract
Background
The integration of Artificial Intelligence (AI) in healthcare is revolutionizing patient care and clinical practice, enhancing efficiency, accuracy, and accessibility. However, it has also sparked concerns among nursing students about job displacement, reliance on technology, and the potential loss of human qualities like empathy and compassion, to this date, there is no established scale measuring the level of fear, especially among nursing students.
Methods
The current study employed a cross-sectional design, involving a total of 225 Saudi nursing students enrolled in a nursing college. The scale's construct, convergent, and discriminant validity were evaluated using exploratory factor analysis (EFA) and confirmatory factor analysis (CFA).
Results
A comprehensive review of the literature addressing fear of AI guided the development of the Fear Towards Artificial Intelligence Scale (FtAIS). An initial pool of items was subjected to a content validity assessment by an expert panel, which refined the scale to 10 items categorized into two dimensions: job issues and humanity. The two-factor structure was responsible for 73.52% of the total variance. The scale items' reliability was evaluated using Cronbach's alpha coefficient, yielding a value of 0.803. The reliability coefficients for the two subscales, job issues, and humanity, are 0.804 and 0.801, respectively. The confirmatory factor model demonstrated a good model fit. The scale's convergent and discriminant validity were both confirmed.
Conclusion
The FtAIS is a rigorously developed and validated tool for measuring nursing students' fears toward AI. These findings emphasize the need for targeted educational interventions and training programs that could mitigate AI-related fears and prepare nursing students for its integration into healthcare. The scale offers practical applications for educators and policymakers in addressing AI fear and fostering its confident adoption to enhance patient care and healthcare outcomes.
1. INTRODUCTION
In an era characterized by remarkable technical progress, there is a growing focus on Artificial Intelligence (AI), an autonomous technology that raises questions regarding its potential to positively transform our lives. The current landscape of AI offers a diverse range of potential and applications. Certain AI systems have already demonstrated capabilities that surpass those of human specialists. Furthermore, these systems are expected to further enhance their optimization soon [1]. The use of advanced technologies in the global healthcare system has garnered significant attention due to its ability to provide high-quality healthcare services. The notion of AI reaching a level comparable to human intelligence evokes fear in certain individuals. Indeed, there is a widespread desire among individuals for machines to assume the responsibilities of monotonous and repetitive tasks that do not necessitate extensive cognitive processing [2]. Furthermore, it is not anticipated that machines will be able to undertake tasks that require human qualities such as empathy, compassion, or autonomous decision-making [3]. Hence, if AI were to undergo further development and acquire complete capabilities for self-teaching and self-awareness, the fear surrounding this advancement would probably intensify [4]. Throughout the course of human history, there has consistently existed a prevalent fear of emerging technologies. Brosnan [5] contended that the prevailing fear of advantageous technology might be characterized as irrational, a sentiment that has been observed to be pervasive in contemporary society as well as throughout history.
1.1. Healthcare, Nursing, and the Fear of AI
The integration of AI in healthcare and nursing care is transforming the landscape of patient management and clinical practice [6-8]. The application of AI in the field of nursing has been observed in several areas, such as the examination of electronic nursing records, the provision of clinical decision support by analyzing pressure sores and safety hazards, the utilization of nursing robots, and the optimization of scheduling [9-12]. The potential benefits of AI in nursing include improved diagnostic precision, optimized workflow, and improved patient outcomes [13, 14]. Despite the recognized benefits of AI, significant gaps exist within nursing education on understanding and application of AI technologies. Most nursing students report limited knowledge of AI and hence anxiety and fear may develop to its use in practice [15, 16]. This knowledge gap is important, as it will be difficult for students to accept AI technologies or adopt these tools for future practice [15, 17]. Technophobia is an irrational fear or anxiety related to advanced technology. It has now emerged as a big obstacle to the acceptance and integration of novelties like AI in healthcare and educational settings [18]. Furthermore, these fears related to AI are often linked with other concerns about job security or the ability of AI to replace human jobs within healthcare [19-21]. Chaibi and Zaiem [22] highlighted that resistance to the adoption of AI is usually based on the fear of healthcare professionals that they will lose their jobs to automated systems that can perform autonomously. This fear is not unfounded, as several studies have shown that many healthcare workers are concerned about the impact of AI on job security and professional identity erosion. For example, a systematic review undertaken by O’Connor, Yan [23] indicates that even though AI assures decision enhancement in decision-making and thus treatment decisions, the involved risks are massive. This includes its ability to automate some roles traditionally set aside for health professionals, thereby having undertones of compromising patients' results. The perception of AI replacing human interaction in health care raises ethical concerns about the quality of delivered care. A human touch would imply the use of empathy, compassion, and emotional support which are the main components of nursing practice [24, 25], while AI can enhance the efficiency and decision-making process of nursing, based on data availability, it cannot mimic the emotional finesse that goes with nursing care [25].
1.2. The Impact of Fear Related to AI
A fear of technology, or technophobia, can cause serious psychological problems and seriously impair daily functioning [26]. Including potential implications for one's professional pursuits. Physical symptoms such as headaches, exhaustion, heart palpitations, perspiration, and anxiety are indicative of the presence of technophobia [27]. The mental manifestations of technophobia include individuals' fears over the sharing of information and their anxieties surrounding engagement with technological advancements [28]. Anxiety and stress among nurses have been linked to healthcare errors that could have fatal consequences [29]. However, nurses' stress levels rise, and the number of mistakes made while caring for patients rises when they are exposed to new technology and suffer from technophobia [30]. Nursing students represent the prospective cadre of professionals that will comprise the future nursing workforce. A significant number of nursing students experience difficulty managing their anxiety or fear levels when utilizing technology in clinical environments [31]. The failure to address technophobia among nursing students may have detrimental consequences for their clinical performance and perhaps result in irreversible impairment of patients' well-being [32]. Hence, assessing the extent of fear towards artificial intelligence among nursing students can aid in identifying training approaches aimed at mitigating such concerns and enhancing healthcare outcomes in subsequent periods. Multiple studies have been conducted to investigate technophobia among various populations, including the general public [33], patients [34], instructors [35], health professionals [36], and nurses, including nursing students [37]. Nevertheless, the primary objective of these investigations has been to ascertain the extent of computer phobia. A survey revealed that 65% of nurses express fears over potential displacement by AI technology in the future [38]. According to a recent survey conducted among 675 nurses in the United States, 30% of the participants were aware of the utilization of AI in clinical nursing practice, while 70% had limited or no understanding of the technology employed in AI [39]. Advancements in AI technology in nursing are typically met with cautious enthusiasm [40]. The utilization of AI technology may give rise to unforeseen repercussions that possess the potential to adversely affect the nursing profession and the fundamental objectives of nursing practice. One potential concern is the possibility of AI perpetuating or systematically incorporating pre-existing human biases into its systems [41]. The utilization of AI tools by nurses can lead to significant and immediate unintended outcomes, which align with the concerns raised by O'Keefe-McCarthy [42] in their analysis of the mediating function of technology in the nurse-patient interaction and its subsequent impact on the ethical autonomy of nurses.
1.3. Available Tools Measuring Fear of Artificial Intelligence (AI)
Although AI remains significant in contemporary culture, research primarily concentrates on the development of novel technological advancements rather than assessing the effects they have. To date, the number of available tools specifically designed to measure the effects of AI implementation remains relatively limited within the current literature. One notable standardized instrument for assessing anxiety related to AI was developed by Wang and Wang [16]. Other researchers developed measures of AI attitudes, such as the Attitudes Towards Artificial Intelligence Scale (GAAIS) [43]. In addition, other researchers constructed a tool to measure technophobia [44]. Another aspect of AI was also studied, as Nazaretsky, Cukurova [45] introduced a new tool to assess teachers’ trust in AI. The integration of AI into healthcare has brought about opportunities and challenges, especially for nursing professionals who are central in patient care. As much as AI has immense potential to enhance efficiency and decision-making, it also brings about concerns about job security, ethical dilemmas, and the erosion of human-centered care. Understanding these fears is essential for preparing the future nursing workforce to adapt confidently to AI technologies. As far as we know, there is currently no study that has developed a comprehensive measure to evaluate the extent of fear felt by nursing students related to AI. Therefore, the main aim of this study was to develop and validate a scale to measure the fear felt by nursing students towards AI.
2. MATERIALS AND METHODS
2.1. Study Design and Setting
For this study, a cross-sectional design was adopted. Because it is possible to collect information from a large number of participants in a very short amount of time, the decision to conduct this research using a cross-sectional survey was driven by the feasibility of doing so. A group of students who were enrolled in a nursing institution in Saudi Arabia were the subjects of the research that was carried out among them.
2.2. Sampling and Data Collection
Sample size recommendations have previously relied on basic principles and expert judgment. For instance, common guidelines suggest a minimum of 100 participants [46], while Lee and Comrey [47] classified sample sizes as 50 (very poor), 100 (poor), 200 (fair), 300 (good), 500 (very good), and 1000+ (excellent). Using G*Power software [48], with a standard error of 0.05 and an effect size of 0.2, the minimal required sample size was determined to be 200. To account for potential low response rates, the sample was increased by 20-30%, resulting in a target of 240 participants. Eligible participants were undergraduate nursing students from southern Saudi Arabia who could comprehend written information, communicate effectively, and have time to participate. Students with pre-existing mental health issues were excluded. Participation was voluntary, and informed consent was obtained. Data collection occurred via Google Forms from September 25 to October 26, 2023, using a structured survey that required complete responses to avoid missing data. Convenience sampling was employed to recruit nursing students from all academic levels (years 1–4) to explore varying levels of fear of AI across different stages of their education. This approach ensured broad representation and minimized response bias while providing valuable insights into nursing students’ fear of AI.
2.3. Procedures for the Development of Questionnaires
The Fear towards Artificial Intelligence Scale (FtAIS) was developed in two stages: (a) item generation and validation, and (b) psychometric properties assessment [49]. The FtAIS was initially designed in the English language due to the widespread familiarity of nursing students in Saudi Arabia with this language, as well as the fact that English is the language of instruction in all nursing colleges.
2.3.1. Stage 1: Item Generation and Validation
The development of FtAIS involved a series of sequential steps that encompassed the design of items and the assessment of psychometric features [38]. Initially, a pool of potential items was generated based on a thorough review of existing literature on AI-related fears and concerns. The items in the English language were mostly generated by the authors using information from the literature review. According to Morgado et al. (2017), Morgado, Meireles [50] the inclusion requirements for an item were as follows: (a) the item had to be pertinent and aligned with one of the primary theoretical domains, and (b) the item had to possess a straightforward, unambiguous, and precise structure and meaning. Consequently, a comprehensive set of 16 items was identified to assess individuals' fear of artificial intelligence. The pool encompassed all the relevant and potentially valuable elements that had been amalgamated. Following the removal of items that had expressions that were identical to those that were included in other items, the process of evaluation proceeded with the remaining 14 items.
2.3.2. Stage 2: Content Validity
A panel of experts, including a psychiatrist, two specialists in AI, two individuals with expertise in AI technology and product usage, and a psychiatric mental health nurse, reviewed the initial 14-item draft of the scale. Based on their feedback, two items were removed, leaving 12 items for further evaluation. A separate expert panel assessed the items' relevance using a 4-point ordinal scale, and content validity was determined using two criteria: Item Content Validity Index (I-CVI > 0.78) and Scale Content Validity Index (S-CVI > 0.90). Results showed that 83.33% (10 items) met the I-CVI threshold, while two items were excluded for not meeting the standard. The refined FtAIS scale, consisting of 10 items, demonstrated strong overall content validity with an S-CVI score of 0.915.
The FtAIS comprises ten items, each evaluated on a five-point Likert scale ranging from 1 (strongly disagree) to 5 (strongly agree). A student's overall level of fear toward artificial intelligence is calculated by summing their scores across all items, resulting in a total score range of 10 to 50.
2.4. Ethical Consideration
This study was approved by the Institutional Ethical Committee (Approval Number-ECM#2023-2907). Informed consent, including details about the current study, was obtained from all the participants. Participation was voluntarily. Anonymity and confidentiality of the responses were ensured for the students. Data collection and storage were done on a secure platform to maintain ethical compliance, and only aggregated results were presented to keep the identity of the participants masked.
2.5. Statistical Analysis
To ensure that there were no mistakes made during transcription, the researchers used a double-entry data method. Data was analyzed using SPSS, which stands for Statistical Package for the Social Sciences, version 25.0, and using the Analysis of Moment Structure (AMOS) software (version 21.0). The data file was randomly split into two equal datasets. The initial dataset, comprising 113 participants, was utilized for reliability analysis, whereas the subsequent dataset, consisting of 112 participants, was employed for exploratory factor analysis (EFA) and confirmatory factor analysis (CFA). The obtained data was summarized and analyzed using descriptive statistics, which included measures such as the average, standard deviation, and corrected item-total correlation. Internal consistency approaches were employed to evaluate the reliability of the FtAIS. The coefficients were estimated and deemed satisfactory if they exceeded 0.70 [51]. Furthermore, the application of exploratory factor analysis (EFA) made it easier to eliminate any components that were included on the scale that were deemed to be insufficient. The component factor was extracted to determine whether or not any of the components had a latent root that was different from the one that was intended. To evaluate the construct, convergent and discriminant validity, exploratory factor analysis (EFA), and confirmatory factor analysis (CFA) was utilized. These analyses included the computation of the composite reliability (CR) of the scale as well as the average variance extracted (AVE).
3. RESULTS
3.1. Participants of the Study
In this study, the average age of male participants was found to be 20.62±1.63 years, while the average age of female participants was found to be 20.45±1.68 years. As seen in Table 1, a total of 225 students, which represents 93.75 percent of the population that was sampled, participated in this study.
Study's Variables | Frequency | Percentage (%) | Mean ±SD |
---|---|---|---|
Age (year) | - | - | Male 20.62±1.63 Female 20.45±1.68 |
Gender | - | - | - |
Male | 124 | 55.11% | - |
Female | 101 | 44.89% | - |
Year level | - | - | - |
1st year | 54 | 24.00% | - |
2nd year | 57 | 25.33% | - |
3ed year | 56 | 24.89% | - |
4th year | 58 | 25.78% | - |
Have you ever participated in a workshop or course that was related to the application of artificial intelligence in nursing or health care? | - | - | - |
Yes | 189 | 84.00% | - |
No | 36 | 16.00% | - |
3.2. Psychometric Properties Assessment
Reliability was assessed by the utilization of internal consistency. The assessment of construct, convergent, and discriminant validity was conducted through the utilization of exploratory factor analysis (EFA) and confirmatory factor analysis (CFA).
3.2.1. The Scale's Reliability Estimates
The purpose of the present study was to construct a standardized measurement scale containing superlative psychometric qualities for the assessment of fear towards artificial intelligence. The corrected item-to-total correlation was the criterion that was used to assess whether or not an item should be excluded from the study [52] as seen in Table 2. This was done to reduce the likelihood of spurious part-whole correlations occurring. Following this, a sequential process was employed to calculate the coefficient alpha, and item-to-total correlations were conducted for each item included in the scale. Items with corrected item-to-total correlations below 0.40 were excluded from the study by the researchers. After making the requisite adjustments, no items had a value less than 0.40. The estimation of reliability typically involves the utilization of the coefficient alpha, which serves as a metric for assessing the internal consistency of a given instrument. The study yielded a coefficient alpha of 0.803. Surpassing
Code | Items containing the Original Numbering | Item-to-total Correlation (corrected) |
---|---|---|
J1 | Q1. I'm afraid that artificial intelligence will eventually take the place of humans. | 0.801 |
J2 | Q3. I'm afraid to work with artificial intelligence techniques. | 0.812 |
J3 | Q4 I'm afraid that employing artificial intelligence techniques may result in a decline in my cognitive abilities. | 0.821 |
J4 | Q6. I'm afraid of developing a reliance on artificial intelligence approaches if I were to use them. | 0.883 |
J5 | Q9. I am afraid of becoming less autonomous if I adopt artificial intelligence techniques. | 0.853 |
H1 | Q2. I am afraid that therapeutic uses of artificial intelligence will cause harm to other people. | 0.720 |
H2 | Q8. I have a fear that artificial intelligence may replace the human empathy and compassion essential in caregiving. | 0.842 |
H3 | Q5. I have a profound fear that one day, artificial intelligence will rule the world. | 0.860 |
H4 | Q7. I am afraid that the expansion of artificial intelligence will reduce interpersonal interaction. | 0.813 |
H5 | Q10. I have a fear that artificial intelligence will interfere with human ethics. | 0.876 |
Item | Job Issues | Humanity |
---|---|---|
Q1. I'm afraid that artificial intelligence will eventually take the place of humans. | 0.751 | - |
Q3. I'm afraid to work with artificial intelligence techniques. | 0.650 | - |
Q4 I'm afraid that employing artificial intelligence techniques may result in a decline in my cognitive abilities. | 0.791 | - |
Q6. I'm afraid of developing a reliance on artificial intelligence approaches if I were to use them. | 0.701 | - |
Q9. I am afraid of becoming less autonomous if I adopt artificial intelligence techniques. | 0.597 | - |
Q2. I am afraid that therapeutic uses of artificial intelligence will cause harm to other people. | - | 0.902 |
Q8. I have a fear that artificial intelligence may replace the human empathy and compassion essential in caregiving. | - | 0.852 |
Q5. I have a profound fear that one day, artificial intelligence will rule the world. | - | 0.871 |
Q7. I am afraid that the expansion of artificial intelligence will reduce interpersonal interaction. | - | 0.893 |
Q10. I have a fear that artificial intelligence will interfere with human ethics. | - | 0.807 |
the minimum criterion of 0.70 as proposed by Hair and Black [53]. The reliability coefficients for the two factors, namely humanity (0.801) and job issues (0.804), indicate their respective levels of reliability. The acceptable reliability level for FtAIS suggests its possible use in different contexts and contributes to the broad research efforts toward understanding and addressing the consequences of AI within professional and educational contexts such as fear of AI.
3.2.2. Construct Validity of the FtAIS
Exploratory factor analysis (EFA) was performed on the ten-item scale to further validate the structure that lies behind it. The FtAIS's factor structure was determined using exploratory factor analysis (EFA) on a pool of 10 items. The Promax rotation approach was employed to investigate prospective factor structures. The oblique rotation method is expected to yield a higher degree of simplicity in the structure, as the components under investigation are truly correlated [54]. The adequacy of the sampling was evaluated using the Kaiser-Meyer-Olkin and Barlett tests before analyzing the findings of EFA. The Kaiser-Meyer-Olkin (KMO) test and Bartlett’s sphericity test were performed to assess if the data was eligible for exploratory factor analysis. The findings revealed a KMO value of 0.807, which is greater than 0.7. Additionally, Bartlett's sphericity test chi-square value (χ2) was 1814.464, and the p-value was less than 0.001. This indicates that Bartlett's sphericity test value reached a significant level, thereby showing that these items were eligible for factor analysis. Oblique rotation with minimum residuals was utilized in the exploratory factor analysis, which was based on the correlation matrix. This was the second use of the technique. Only factors with an eigenvalue greater than 1 were kept [55]. According to the findings, there were no items that needed to be removed. At the end, ten items were kept. Table 3 displays the outcomes of the exploratory factor analysis. As an additional point of interest, the total variance explained reached 73.52% following the extraction of two components.
3.2.3. CFA (Confirmatory Factor Analysis)
The CFA has been employed to assess the dimensionality of FtAIS, alongside the EFA. Confirmatory factor analysis (CFA) was employed to assess the validity of the two-component structure of the scale. The findings of the study confirmed that the two-factor structure was the most suitable fit for the ten-item scale. Fig. (1) illustrates the path map that provides the confirmatory factor analysis (CFA) results for the ten items of FtAIS. The route diagram illustrates the factor loadings. The results indicated that the ten-item scale would be most appropriate for a two-factor framework. Fig. (1) contains the route map that illustrates the CFA of the standardized regression for the ten items that are included on the FtAIS. In the route diagram, the standardized regression coefficients, which are sometimes referred to as factor loadings, are displayed for each of the observable variables (represented by rectangles) as well as the latent variables (indicated by circles). Furthermore, the amount of variance (R2) that is displayed is an indication of the degree to which the latent variables explain the observable variables. The construct validity of the two-factor structure retrieved from EFA was evaluated using the maximum likelihood estimation approach in CFA. Schermelleh-Engel, and Moosbrugger [56] introduced benchmarks to determine a satisfactory fit, which served as guidelines for assessing the appropriateness of model fit. Among these benchmarks are the following: (a) In an ideal scenario, factor loadings should be greater than a critical ratio (CR) of 1.96. It is recommended that the index of relative chi-square (χ2/df) not fall below the value of 5. (c) The comparative fit index (CFI) and the normed fit index (NFI) should both be at least 0.85 to be considered optimal. In addition, it is recommended that both the goodness of fit index (GFI) and the adjusted goodness of fit index (AGFI) be at least 0.86. (e) The root mean square residual (RMR) and the root mean square error of approximation (RMSEA) should preferably be no less than 0.08 to be considered acceptable.
All of the factor loadings were found to be between. 0.70 and 0.85. A total of ten items were included in FtAIS. The fit indices for this scale were as follows: χ2 = 2752, df = 1400, χ2/df ratio = 1.96, p <0. 001. The FtAIS fit indices for the two-factor analysis, as seen in Table 4, exhibit strong evidence of the model's adequacy in representing the underlying constructs. Overall, these fit indices collectively indicate that the two-factor model of the FtAIS provides a robust and accurate representation of individuals' fear of artificial intelligence, supporting its validity for measuring these constructs effectively. This ensures that educators, researchers, and policymakers can accurately identify the levels and dimensions of fear toward AI, enabling targeted interventions.
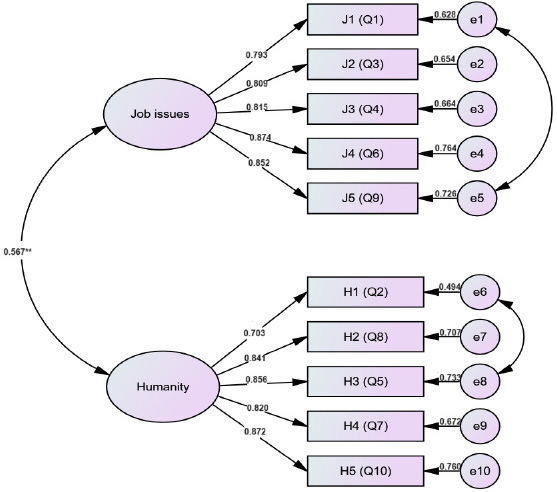
A model for fear towards artificial intelligence scale.
FtAIS Fit Indices | FtAIS Two-factor Value | Standard Value |
---|---|---|
Comparative Fit Index (CFI) | 0.92 | >0.85 |
Adjusted goodness of fit index (AGFI) | 0.86 | >0.85 |
Root Mean Squared Error (RMSEA) | 0.07 | <0.08 |
Normed Fit Index (NFI) | 0.91 | >0.85 |
Standardized root mean square residual (RMR) | 0.05 | <0.08 |
3.2.4. Discriminant and Convergent Validity
The Exploratory Factor Analysis (EFA) was initially used to evaluate the ability of the FtAIS to differentiate between different factors and to determine if it measures the same construct as other related measures. Afterward, a correlation matrix approach was used to measure the accuracy of the FtAIS. Convergent validity assesses if the correlation analysis of a specific theoretical framework produces a statistically significant non-zero outcome, which justifies the need for further examination of discriminant validity.
The evaluation of discriminant validity was performed using the confidence interval method, as suggested by Anderson and Gerbing [57]. The correlations between the two dimensions are outlined in Table 5. The two dimensions displayed a significant relationship, indicating a common FtAIS construct between them. Nevertheless, the confidence ranges for the pairwise correlation between these two variables do not include the value of 1.00. Hence, the multiple-item measures exhibited discernible validity.
Main Subscales | Items (N) |
Convergent Validity | √AVE | Discriminant Validity | ||
---|---|---|---|---|---|---|
AVE | CR | Scale Correlation Matrix | ||||
Job issues | 5 | 0.530 | 0.843 | 0.728* | 1.000 | 0.563* |
Humanity | 5 | 0.542 | 0.833 | 0.736* | 0.563* | 1.000 |
When referring to a particular construct, the term “convergent validity” describes the degree to which items for that construct have a high level of shared variance. On the other hand, discriminant validity is a term that describes the degree to which a certain construct is unique from other comparable constructs. Measures such as composite reliability (CR), average variance extracted (AVE), maximum shared variance (MSV), and average shared variance (ASV) are utilized in the process of evaluating convergent and discriminant validity.
ASV stands for the average amount of shared variance among constructs, CR stands for the reliability of the composite construct, AVE stands for the average amount of variation explained by the items that are contained within the same construct, MSV stands for the maximum amount of shared variance among constructs, and ASV stands for the average amount of shared variance among constructs. A determination of convergent validity was made by determining the Average Variance Extracted (AVE) of each construct and determining the correlation between that construct and other constructs. Convergent validity was considered to be confirmed when the AVE value exceeded 0.50 [55].
To demonstrate discriminant validity, the mean square variance (MSV) and the average shared variance (ASV) for each and every construct must be lower than the average variance extracted (AVE) [55]. The fact that the average scores for all dimensions on the FtAIS were greater than 0.50 and higher than the correlation with other items is evidence that the scale possesses convergent validity. The fact that the MSV and ASV values were found to be lower than the AVE values for every scale construct is evidence that the FtAIS possesses discriminant validity (Table 5). If the square root of the average variance extracted (AVE) for a component is higher than the Pearson correlation coefficients of other components, this indicates that the component has a reasonably high level of discriminant validity.
4. DISCUSSION
The purpose of this study was to create and validate a tool for evaluating the level of fear that nursing students feel toward artificial intelligence. The creation and validation of FtAIS required a comprehensive review of the current body of knowledge. Exploratory Factor Analysis (EFA) was performed, and the results of that analysis showed the factor structure of the FtAIS. The FtAIS is made up of ten different items that collectively evaluate two different factors: job issues and humanity. On a Likert scale with five points, each item is evaluated from one (strongly disagree) to five (strongly agree). A student's level of fear over artificial intelligence can be determined by adding up their scores in each of these areas, which can range anywhere from ten to fifty. More fear about artificial intelligence is indicated by a higher score. According to Cronbach's alpha, the scale has an acceptable level of reliability (0.803), and it also possesses great convergent validity, which makes it an efficient scale for evaluating fear concerning artificial intelligence. It is important to note that the R values of the scale are greater than 0.30, which indicates that no items should be removed from consideration. According to the results of the CFA, the 10-item scale would be best served by a structure consisting of two factors. Nursing students harbor various fears regarding the integration of AI in nursing related to fear concerning job issues (subscale 1).In line with other studies, it has been found that the advent of technologies, such as health information technology, has facilitated nurses` continuous surveillance of patients in recent decades [29]. Nurses who hold this perspective may express opposition against the implementation of AI in the healthcare sector due to concerns regarding the potential obsolescence of their profession [58]. In addition, the literature supports the notion that nursing students express fear about the potential for AI to replace human roles (Q1) and nursing students exhibit fear of working with AI techniques (Q3). In line with Castagno and Khalifa's [19] study findings, it was found that 10% of participants had worries about AI replacing them at their jobs. AI technologies may eventually replace some jobs, perhaps leading to a loss of meaning as human activity is automated and computerized [59, 60]. It was also found that a significant proportion of students (24%) perceived AI as a threat to the healthcare sector and held a negative attitude regarding it [60]. These findings are significant, as they highlight the need for educational programs that not only enhance technical competencies but also address the psychological aspects of AI adoption [13]. Additionally, there is a prevailing fear among nursing students that employing AI techniques may lead to a decline in their cognitive abilities (Q4). This could be due to AI is capable of executing tasks that require human cognitive abilities, such as speech recognition, picture perception, decision-making, and language translation [61]. The nursing curricula should be complemented with training in critical thinking and decision-making, using AI as a tool. This will further enhance a sense of autonomy and competence in the use of AI and make them use of technology as a tool for their professional development [62].
Moreover, nursing students express fears about developing a reliance on AI approaches (Q6), becoming less autonomous in their practice (Q9) and AI will rule the world (Q5). In comparison, it has been observed that AI-assisted systems are expected to carry out distinct functions such as test referrals and patient screening, as well as provide recommendations for prospective therapeutic interventions and gradually achieve greater autonomy [63]. Regarding the therapeutic uses of AI, nursing students fear that AI may cause harm to patients (Q2). Similar to the findings of Ahmed, and Spooner [64] in which safety issues were considered a barrier to AI integration into health care. Additionally, nursing students also have concerns about the potential reduction in interpersonal interaction with the expansion of AI (Q7). According to Loncaric, Camara [65] there will be a loss of the holistic medical approach as healthcare shifts towards data analytics and away from patient interactions. Imtiaz and Khan [59] express concerns about the potential consequences of incorporating robot caretakers since they argue that such a development may lead to a displacement of human-to-human interactions, like how online interactions have supplanted face-to-face socializing. As a result, nursing education should focus not only on technical training but also on the development of interpersonal skills and emotional intelligence [66]. By reinforcing the importance of human connection in caregiving, educators can help students appreciate the unique contributions they make to patient care that AI cannot replicate.
Lastly, nursing students fear that AI may interfere with human ethics (Q10) and fear that artificial intelligence may replace the human empathy and compassion essential in caregiving(Q8). Similarly, systematic review research [64], found a notable ethical problem that serves as an obstacles to the integration of AI in the healthcare sector. In addition, Mehta, Harish [67] found that students believe AI will present new ethical and social dilemmas. A total of 39 studies were examined in their study specifically addressed these ethical barriers. The most significant themes within the context encompassed privacy, trust, consent, and conflicts of interest. According to this perspective, the ethical implications of human-machine relationships are inherently detrimental, as they only provide a deceptive semblance of friendship, affection, and interaction with others. A notable pattern observed in the nursing literature on AI technology is the inclination to perceive technology as a means of depersonalization, dehumanization, and as being fundamentally in conflict with the principles of compassionate care [19]. In addition, Verma [68] argues that nursing programs should incorporate AI ethics into their curricula, with a focus on AI transparency. Teaching cyber ethics and appropriate use of artificial intelligence is necessary for nursing education to stay up with the changes caused by the widespread use of informatics and related technology in professional life [14].
4.1. Implications
This study has relevant implications for nursing practice, education, and healthcare policy. Efforts need to be directed at reducing the fear of AI among nursing students so that they can adopt and integrate technology into the clinical environment without losing sight of or focusing on patient-centered care. Nursing curricula should thus include AI-focused training that promotes technical competence, critical thinking, and decision-making while reminding graduates of the irreplaceable place of human compassion in caregiving. Through using practical, hands-on training with AI tools in simulated or controlled environments, students would further experience the benefits of AI without feeling threatened by its complexities. The FtAIS can be used to identify specific concerns and tailor interventions to reduce fear and foster confidence. Moreover, educational programs should ensure that students view AI as a complementary tool rather than a threat to their professional roles. At the healthcare policy level, strategies must balance technological efficiency with preserving the interpersonal aspects of care, such as promoting emotional intelligence and ethical decision-making. For healthcare systems, this means fostering trust in AI while reinforcing empathy and human interaction as core values, creating environments that support the ethical and effective integration of technology. These implications underpin a more holistic approach to embedding AI in healthcare, where innovation in both technology and human connection is vital to deliver quality care.
5. LIMITATION
Various limitations to this study should be considered. First, participants' responses concerning their fear of AI may be influenced by potential biases such as social desirability bias, which could be introduced using self-reported scales. Second, cultural factors specific to the sample, such as societal attitudes toward technology and education systems, may have influenced the findings, limiting the generalizability of the results to other cultural contexts. Third, a robust psychometric validation procedure confirmed the development of the scale. However, there is potential for overlap between factors in the psychometric validation process. Future research utilizing translation, cross-cultural validation, and alternative approaches is needed to thoroughly evaluate the scale and address these limitations.
CONCLUSION
This study's primary contributions involve the creation of a novel scale to assess the level of fear nursing students experience toward AI. The emergence of the FtAIS signifies a notable advancement in the theoretical progression about the use of AI. It represents a significant advancement in the theoretical understanding of students' fear of AI technology. With its two distinct subscales focusing on job-related concerns and impacts on humanity. The scale's ten items demonstrate robust reliability and validity, making it a valuable tool for researchers and practitioners alike. Additional approaches, such as cross-sectional research, can be valuable for examining a representative subset of data related to job-related concerns and the humanity of utilizing AI in nursing care. By employing FtAIS in the nursing context, educators and researchers can effectively assess the extent to which nursing students experience fear related to AI adoption in clinical practice. This assessment can provide valuable insights into potential barriers to AI implementation in nursing care delivery, guiding educators in developing targeted educational interventions to address students' fears and enhance their comfort level with AI technologies. Furthermore, understanding nursing students' fears of AI can inform organizational strategies aimed at fostering a supportive environment for AI integration, promoting acceptance and collaboration among healthcare professionals. Policymakers are encouraged to develop strategies that balance technological efficiency with the preservation of human compassion in care delivery. Investments in AI adoption need to be coupled with initiatives that promote ethical decision-making, emotional intelligence, and interdisciplinary collaboration. By fostering environments that emphasize both technological proficiency and the irreplaceable value of human connection, all stakeholders can ensure that AI will find its successful integration into healthcare ultimately benefit patient outcomes, and support the next generation of healthcare professionals.
AUTHORS CONTRIBUTION
It is hereby acknowledged that all authors have accepted responsibility for the manuscript's content and consented to its submission. They have meticulously reviewed all results and unanimously approved the final version of the manuscript.
LIST OF ABBREVIATIONS
FtAIS | = Fear Towards Artificial Intelligence Scale |
EFA | = Exploratory factor analysis |
CFA | = Confirmatory factor analysis |
CR | = Composite reliability |
AVE | = Average variance extracted |
KMO | = Kaiser-Meyer-Olkin |
MSV | = Maximum shared variance |
ASV | = Average shared variance |
ETHICS APPROVAL AND CONSENT TO PARTICIPATE
The study obtained approval from the King Khalid University Ethical Committee (ECM#2023-2907), Saudi Arabia.
HUMAN AND ANIMAL RIGHTS
All human research procedures followed were in accordance with the ethical standards of the committee responsible for human experimentation (institutional and national), and with the Helsinki Declaration of 1975, as revised in 2013.
CONSENT FOR PUBLICATION
All participants in this research supplied their consent after receiving enough information.
AVAILABILITY OF DATA AND MATERIALS
The data and supportive information are available within the article.
ACKNOWLEDGEMENTS
The authors would like to express their gratitude to the participants of this study.